Will machines take over mental health care?
Artificial intelligence has a lot to offer to patients struggling with their mental health, but experts say we shouldn’t expect it to replace humans
Dana Najjar • February 6, 2019
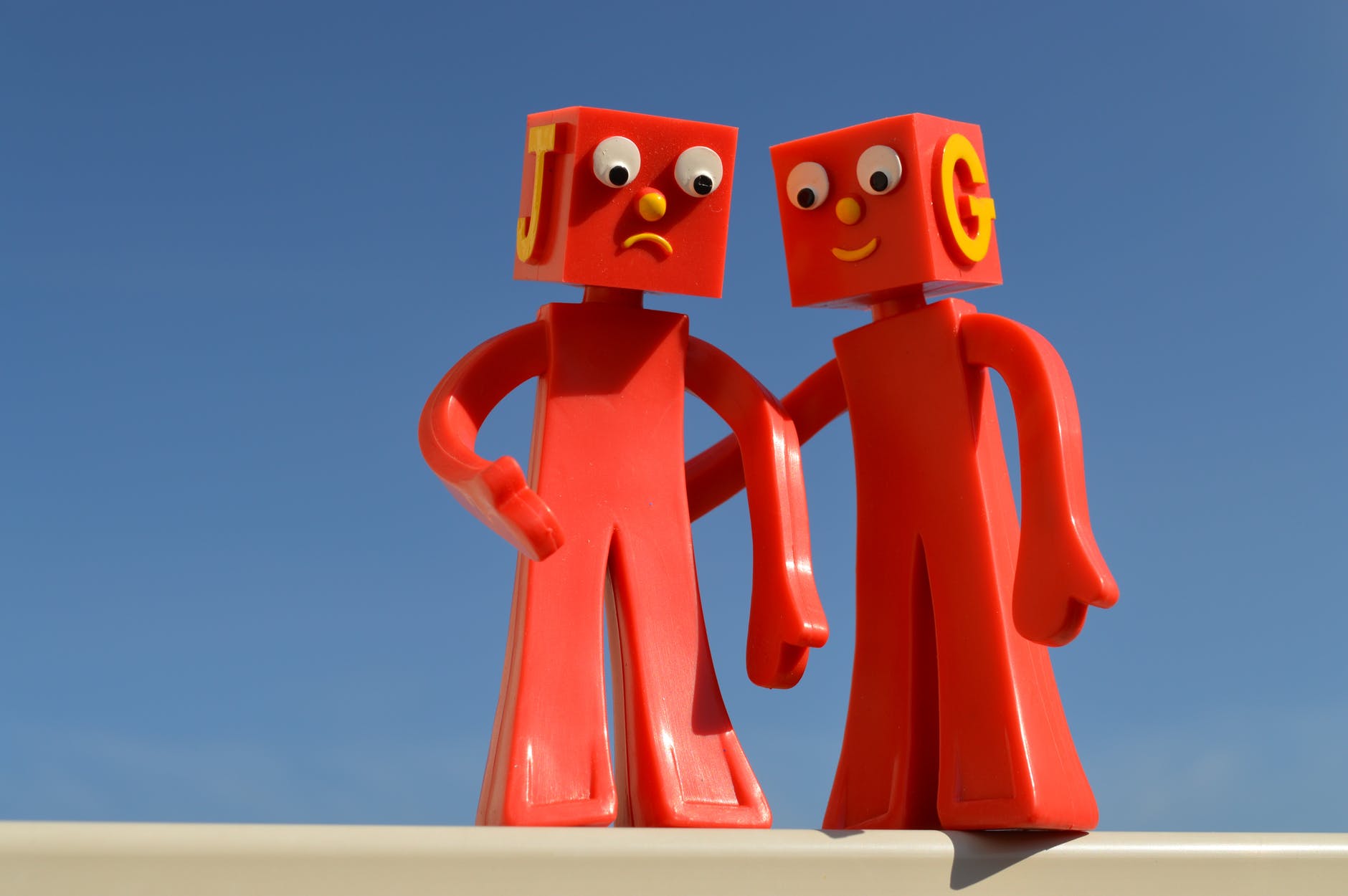
The machines are here, and they want to help. But we may not be ready for them. Source: Creative Commons
“Philosopher, Life Coach & friend,” reads Woebot’s Twitter profile. “Studying humanity at the school of life.” And if you failed to glance at the little yellow robot’s avatar, you just might mistake it for a person. But Woebot is a bot.
More specifically, it is a conversational phone app designed to coach users through episodes of anxiety or depression. Woebot provides a personalized virtual coaching based in cognitive behavioral therapy, for $39 a month and available any time, day or night. It’s one of many such tools that have emerged over the past few years that throw the promise of technology at the growing mental health crisis we face.
“We wanted to make evidence-based mental health care tools radically accessible globally,” says Athena Robinson, chief clinical officer at Woebot. “There are millions of people across the world who do not get access to the mental health care that they need.”
And though the technology is very new, preliminary studies suggest that it works: A 2017 study co-authored by Woebot’s founder Alison Darcy found that Woebot users showed a “significant reduction” in anxiety and depression compared with a control group who only had access to coaching books. “Conversational agents appear to be a feasible, engaging, and effective way to deliver CBT,” the authors concluded.
Chatbots aren’t the only smartphone tools out there for mental health care. Ginger is an application that leverages artificial intelligence and machine learning, but ultimately connects users with therapists who are able to provide customized care.
Regardless of their specific application, all these tools are based on machine learning, or the science of teaching computers to spot trends and make predictions in data without being explicitly programmed to do so, and in a way that improves over time. Though still a burgeoning branch of artificial intelligence, machine learning has already insinuated itself into many aspects of our daily lives: When Amazon makes recommendations on what you should buy next, or Google Maps suggests the ideal commute, that’s machine learning in action. The same goes for Siri, Alexa or any other voice recognition tool that gets smarter the more people use it.
At its most basic level, machine learning operates by feeding a computer algorithm lots of data and allowing it to learn from them. For example, medical researchers might give a computer algorithm images of different tissue samples from patients whose diagnoses are known. The algorithm is told which tissue samples are cancerous, and which are healthy. These initial data are known as a training set, since the algorithm will use them to learn how to recognize cancerous tissue on its own. Eventually, it will be able to categorize new images by itself.
Machine learning in mental health care isn’t restricted to smartphone apps. Claire Gillan at Trinity College Dublin is trying to predict individual patients’ responses to treatments in order to help clinicians recommend personalized care. At present, only 40 percent of patients respond to the antidepressant that they are prescribed, Gillan explains. “There’s no need to have someone suffer another 3 months trying an antidepressant that’s not going to work for them,” she says. “If you could identify who’s going to be sensitive [to an antidepressant], you could fast-track them to a treatment that’s going to work for them.”
Does this mean that we’re moving toward a world where clinicians will be redundant, and machines will do the work of diagnosing, counseling and prescribing treatment? Almost certainly not.
“That’s one of the biggest misunderstandings that we’re working really hard to rectify,” says Woebot’s Robinson, who is herself a clinician and stresses the importance of a human connection when it comes to mental health care. “Woebot is not meant to be a replacement, but it’s meant to get help to people who can’t get to a physician or who may not need that intense level of expertise that a physician can offer.”
Gillan agrees. “The relationship that you have and the conversations that you have in a doctor’s office are so important,” she says.
Others are also hard at work trying to make sure that we don’t mistake these shiny new tools for a global panacea. Sherry Turkle, founder and director of the MIT Initiative on Technology and Self, argues that our increased dependence on smartphones, tablets and computers comes at a very high cost, even when the devices help us cope with mental health issues. By steering us away from being alone with ourselves, and from being alone with others, Turkle says they limit our capacity for introspection and empathy. She is particularly wary of bots that we may come to view as almost human. “These robots can perform empathy in a conversation about your friend, your mother, your child or your lover, but they have no experience of any of these relationships. Machines have not known the arc of a human life,” she wrote in an op–ed for The New York Times earlier this year.
There are other things to worry about besides losing the crucial doctor-patient relationship. Machine learning is not infallible — the quality of the predictions and recommendations depends on the data used in the training set, and data can be biased. Think back to the example of cancer detection: If all the training images are white skin, then it is highly unlikely that the algorithm will learn to correctly identify skin cancer in black patients. There are many other real world examples of machine learning failures: Self-driving cars have accidents, chatbots are easily manipulated and facial recognition can be fooled by a mask. “There are instances where algorithms just fail for really dumb reasons,” Gillan says. “And the kind of idiotic things an algorithm will suggest, a clinician will know are completely wrong.”
In the case of chatbots aiming to reach marginalized global communities, the biases in the data could be cultural, making it hard for the bots to discern signs of crises in non-Western users.
In addition to its technical shortcomings, this new technology also raises a host of ethical and legal issues that physicians, governments and other regulatory bodies have not yet had the time to resolve. “A lot of these companies that are doing stuff with chat bots, they’re sort of calling them coaches or assistants, but they’re really getting into that domain of mental health care,” says David Luxton, a psychologist who focuses on the ethics of artificial intelligence use in medicine. “It raises all these ethical issues, and potentially legal issues. They’re advising people on health behaviors, but they’re not doing it from the position of a licensed healthcare provider.”
In other words, who’s to blame if the machines get it wrong? They’re smart, and they’re only getting smarter, but they’re a long way from being perfect. “The data that’s going in could be inaccurate,” Luxton says. “It might have particular biases.” Right now, we don’t have any guidelines in place for dealing with such pitfalls.
What’s more, there’s a lot that researchers don’t understand about the artificial intelligence models they’re building. Luxton calls this the “black box problem,” saying that “artificial intelligence look[s] at massive amounts of data to identify patterns, then it provide[s] some kind of recommendations, but we may not understand how it drives those recommendations because the algorithms are so complex. That could be a potential liability in a legal situation.”
Is it conceivable that, in time, these kinks will be ironed out and give way to near-perfect systems backed by the appropriate regulatory and legal frameworks? Maybe, but it’s far more likely that machine learning tools will never be more than intelligent aids for physicians to draw upon when diagnosing and treating mental illnesses. “It’s not beyond the bounds of possibility that these things could be algorithmically driven,” Gillan says. “But it’s hard to conceive of a world where that’s the case.”
*Correction, Feb. 20: An earlier version of this story incorrectly characterized the app Ginger. It provides customized mental health care via text-based chats with emotional health coaches, as well as teletherapy and telepsychiatry services.
1 Comment
Good morning. Interesting article, like the topic discussed. In my opinion, I think that the work of Coach requires empathy, participation, feeling of having found an ally in interlocutor, all elements that require a human Coach. Surely robots can be a valuable help for people. But I think that, in certain sectors, human relationships between humans are needed.