When code becomes conservation
Artificial intelligence is becoming increasingly common in conservation ecology. Should it be?
Joanna Thompson • February 8, 2021
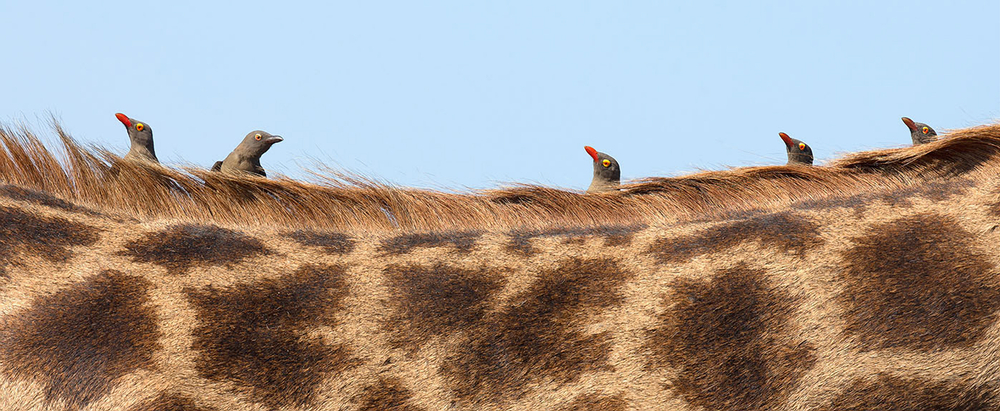
AI is great for identifying giraffes, but it misses hitchhikers like these oxpecker birds. [Credit: Ken Hadfield, Shutterstock | CC BY-NC-SA]
Something was amiss with the giraffes. The animals, which looked healthy in daylight, seemed to sprout strange underarm tumors at night. This bizarre scene was captured by nocturnal camera traps as part of the Snapshot Serengeti project in Tanzania’s Serengeti National Park.
Concerned, the project’s citizen scientists alerted the experts. “They were like, what’s going on with these giraffes?” recalls Meredith Palmer, a behavioral ecologist with Snapshot Serengeti. Careful examination revealed a surprising culprit: dozens of yellow-billed oxpecker birds, which had taken to cuddling in giraffe armpits at night.
Why didn’t researchers notice these freeloaders sooner? The artificial intelligence algorithm tasked with processing camera data missed them entirely.
This is just one example of the limitations of AI in ecology. Increasingly, conservationists and computer engineers are teaming up to train machine-learning algorithms to recognize wildlife. But some researchers worry that this approach will produce inaccurate data, which could lead to misguided or even harmful policy decisions.
Advocates, however, argue that AI’s potential upsides are too good to ignore. They imagine a future where scientists can process camera trap data at lightning-fast speeds, catch poachers with ease and even recognize individual African lions by their roars alone. In the future, this could prove crucial for conservation efforts.
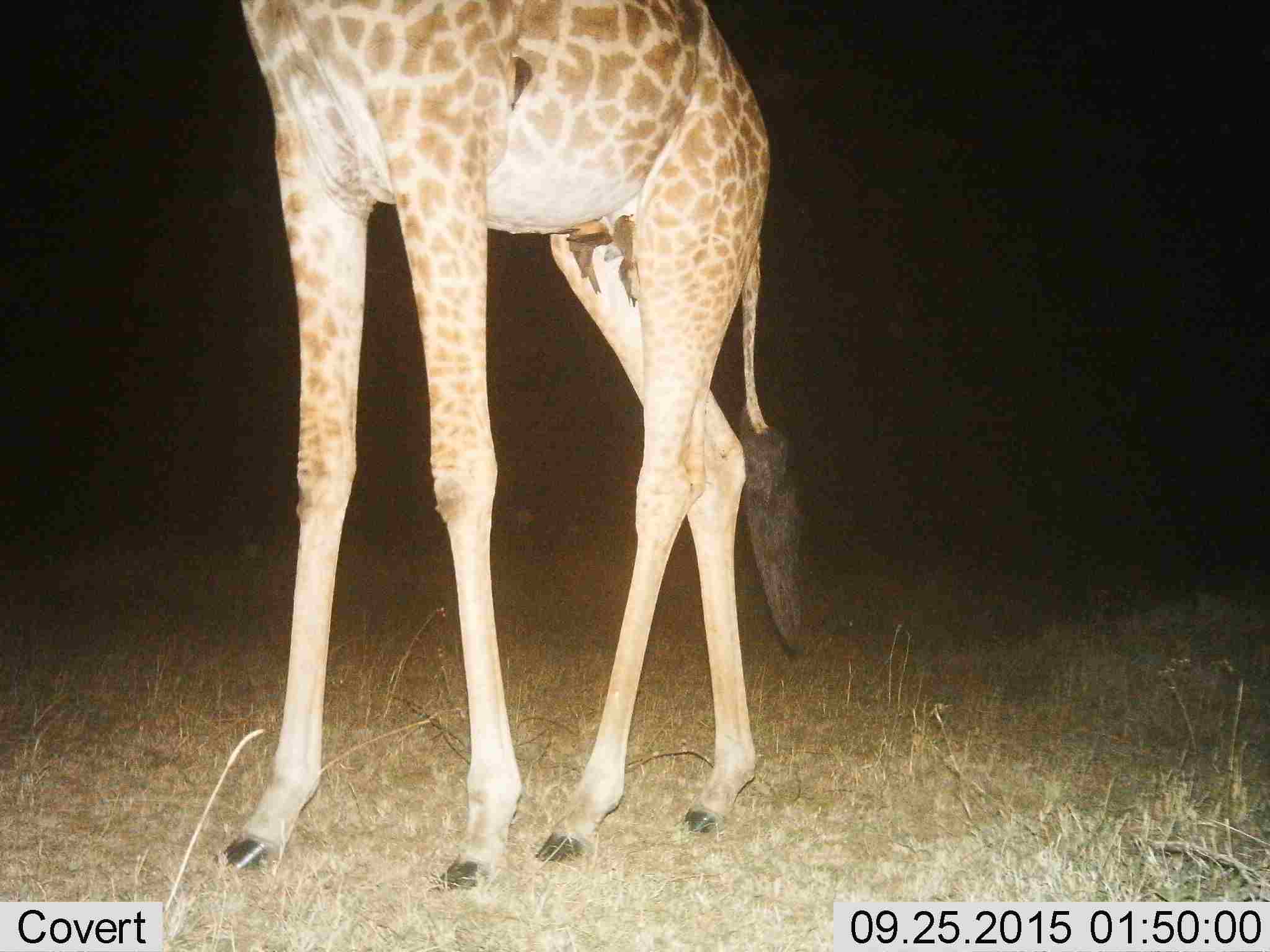
An image of armpit-roosting oxpeckers captured by Snapshot Serengeti’s camera traps.[Credit: Snapshot Serengeti, Zooniverse| CC-BY-4.0]
Victor Anton, CEO of the New Zealand-based company Wildlife AI, has firsthand experience with combining code and conservation. Early in his career as a zoologist, Anton trained an algorithm to recognize the call of the endangered stitchbird. Or so he thought.
As it turned out, most of the sites from which they collected these sounds “had a small creek or river nearby,” says Anton. “The algorithm was picking up the sound of the river, and not the actual bird call.” Fortunately, he caught the error quickly and retrained the program. Botched bird calls might not seem like a big deal, but researchers should be wary of this kind of unintentional bias creeping into their models, says Anton.
Bias in AI trained to analyze humans has already made headlines. In June, Amazon, IBM and Microsoft all halted sales of their facial recognition software to law enforcement after it proved pretty awful at distinguishing between Black individuals. The same pitfall exists in wildlife applications — but unlike humans, birds and giraffes can’t point out the disparity to us. So if, for example, Anton’s original algorithm had been used to establish a stitchbird sanctuary, it would have encompassed a lot of running water and very few birds.
Still, AI may prove essential to conservation ecologists in the long term. Predation researcher Ali Swanson, who worked with Snapshot Serengeti in the past, says that camera traps generate mountains of data. Currently, it would take one person something like seven years of nonstop toil to process all of the information Snapshot Serengeti collects in a single year, according to Palmer. An algorithm, in contrast, is able to do it in less than two weeks with the help of the project’s citizen scientists to double-check the results.
Machine learning also transformed Oxford zoologist Matthew Wijers’s work. Wijers and his team were analyzing lion roars in Zimbabwe to see if different lions had a signature call. Initially, he says, they were looking at individual roar components, like length and tone, “trying to see if those particular variables are actually informative or not. And it turned out they aren’t.”
So they switched tactics. “We thought, let’s try this machine learning approach which takes into account the whole pattern itself,” says Wijers. The AI was able to recognize individual lions with 91.5% accuracy — a massive improvement. It even picked out one individual that had snuck onto the Zimbabwean preserve from neighboring Botswana. The identification implies that there might be geographic variation in lion roars, Wijers says, a prospect that the team would have missed without their algorithm.
Right now, the consensus in the ecologist community seems to be that a blend of artificial intelligence and human oversight is the best path forward for conservation. “There’s a risk of seeing it as a panacea. [That’s why] we look at integrating human and machine interface,” Swanson says.
Meredith Palmer agrees. “It’s worthwhile to have at least one set of human eyes on those pictures,” she says. “I’m terrified that if we switch entirely to AI, I wouldn’t have armpit birds in my life. And it would be that much worse, you know?”
Editor’s Note (Feb. 9 2021): This story was updated to clarify the timeline of Ali Swanson’s involvement with Snapshot Serengeti. We also attributed the estimated time for one human to process a year’s worth of data vs an algorithm to Meredith Palmer.