Catching the next pandemic early: the coronavirus is one example
Machine learning may boost disease hunting, but not everyone’s convinced
Taylor White • April 21, 2020
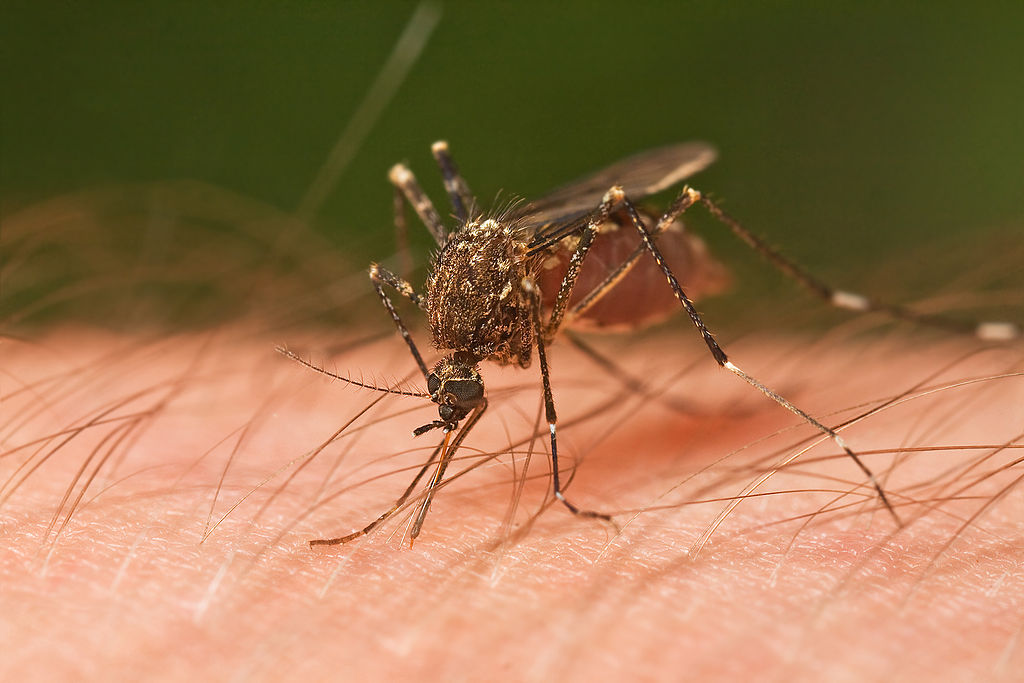
Animal-borne diseases threaten millions of people around the world. [Credit: JJ Harrison/Wikipedia | CC BY-SA 3.0]
An intimidating image of a mosquito, magnified about 1,000 times larger than life, glared from a screen at the front of the room. A cellphone blared, interrupting the rhythmic jazz playing in the background. The ringtone was the Bee Gees’ “Stayin’ Alive.”
The slightly ominous mood matched the topic: the highly infectious animal-borne diseases that threaten hundreds of millions of people around the world. Yet there was no sense of existential dread at a recent talk in New York City; probably because the speaker, disease ecologist Barbara Han, has a surprisingly optimistic take on the ability to better predict human disease outbreaks.
The reason? Artificial intelligence.
Han uses artificial intelligence to forecast zoonotic diseases — animal-borne diseases that spill over to humans — to predict and hopefully prevent future outbreaks. When the model successfully predicts if an animal is a disease carrier, she said, it’s hard to know whether to celebrate over or feel even more anxious about whether an outbreak may be imminent.
“It’s one of those moments where you’re not sure whether to cheer because it’s working or ‘that’s awful,’ like what do we do with that information?” said Han, who works at the Cary Institute of Ecosystem Studies in Millbrook, New York. She spoke recently to an appreciative audience of 70 at the Greene Space, a performance venue in Manhattan.
Companies are already using artificial intelligence to predict disease outbreaks worldwide — and governments and hospitals are looking to them for input. Using data related to global infectious diseases, these elaborate computer models are aimed at predicting when, where, and how animal-borne viruses like Ebola (carried by monkey species) and Zika (by mosquitoes) may break out in human populations and trigger deadly epidemics. BlueDot, based in Toronto, is one artificial intelligence company that does this — and they were the first to identify the coronavirus outbreak in Wuhan about a week before the World Health Organization, U.S. Centers for Disease Control, and China made any official announcements.
The key to these models — and the source of Han’s optimism — is a computing process known as machine learning.
Machine learning is already ubiquitous in our lives. It’s the reason why Netflix knows what shows we’re likely to watch next and why Facebook ads reflect our interests.
Machine learning uses data collected over time to learn and identify patterns, said Dr. Davidson H. Hamer, who studies infectious tropical diseases at Boston University. As the computer collects more data, patterns become easier to recognize. For example, in a clinical scenario, you learn more about certain characteristics or symptoms of a disease over time as you add information from more and more patients to a database, said Hamer.
In disease epidemiology, machine learning is still experimental — though one that’s quickly gaining traction, according to Han.
Han feeds her models data about monkeys and other animals likely to be carrying diseases. With enough information about their diet, location, reproduction rate, and other biological and ecological factors, over time these models can make increasingly accurate predictions about disease reservoirs in animals and where an outbreak could pop up next.
“You map their [the animal’s] distributions, you can figure out where they’re overlapping, how close they are to known outbreaks,” said Han. “Now you’re kind of triangulating what species, where they live, who’s getting sick, what they’re getting sick with, and suddenly the prediction test seems much more achievable.”
It’s easy to see why so many disease trackers are turning to AI for help. Outbreaks of animal-borne plagues occur among humans with distressing regularity. From 2013 to 2016, an Ebola outbreak in West Africa killed more than 11,000 people. The virus was carried by an animal species but still no one knows what animal started the outbreak, said Han. In all, roughly 65% of human infectious diseases originate in animals.
Not all experts are as upbeat about the power of machine learning to avert epidemics. “I’m a little skeptical, to be honest,” Hamer said. He describes how computer models like Han’s are only as good as the data that’s fed to them. When there are gaps in that data, people have to extrapolate what’s missing. “That always worries me because depending on how you make those estimates, you could sort of push your ideas in one direction or another,” he says.
In terms of BlueDot’s machine learning process for predicting the spread of diseases like COVID-19, Hamer thinks BlueDot’s disease-forecasting could be more accurate than Han’s because they use more of a variety of data.
“BlueDot uses a variety of different data sources that go beyond what Dr. Han was using,” says Hamer. “They [BlueDot] look at flight patterns, distribution of vector, the temperature and humidity, and other factors.”
In any case when a completely new outbreak happens, like COVID-19, he says it may be difficult for machine learning to identify or predict this. But once a disease is identified, along with the right mix of datasets, Hamer says it is possible for machine learning to make early predictions of where an outbreak might spread next.
“This [COVID] is a completely new virus and I think it’s emergence is just really hard to predict.” says Hamer, “It’s hard to know when and where it [an outbreak] will happen, but once it starts to happen then you can predict.”
Han acknowledges that it is a challenge to manually input so much data, as well as to keep the algorithm she uses up to date as new research is published about which animals carry which diseases. Even so, she asserts, some of the models have a high accuracy for predicting if an animal is a human disease reservoir.
For example, in her rodent reservoir study, she looked at 2,277 rodent species to predict which species might cause an outbreak. She fed information from about 80% of these rodent species into her computer model — including whether or not the species was a known carrier of a zoonotic infection.
Other traits she analyzed included the size of the species’ geographic range and how they reproduce. Using this data, the model gradually learned how to predict whether a species was a disease carrier based on its specific traits. Then Han tested the model on the remaining 20% of rodent species. The model then predicted these species’ statuses as disease carriers. Based on this test, the model correctly identified the disease status of 90% of the rodent populations, according to an article Han wrote for IEEE Spectrum.
As more data becomes available, Han hopes to make her computer models even more accurate so that they perform in real-time, keeping predictions constantly updated. In April 2019, Han collaborated with NASA Goddard Space Flight Center to build a prototype early-warning system for animal-transmitted diseases.
Predicting the next outbreak, Han said, is like trying to solve a mystery with only a few vague clues. But she wants to put the clues we do have to better use.
“It’s like smoke and whispers. You know it’s there; you know it’s making people sick, but you just still haven’t found the smoking gun,” said Han.
Apr. 21, 2020: This is an updated version of the original article, published on Dec. 9, 2019. This article contains recent information about COVID-19 and BlueDot.
2 Comments
Hi,
Do you know of any company whose machine learning predicted the emergence of COVID19?
Hi Francis, good question! There’s actually an artificial intelligence company called BlueDot that I linked to in this story. BlueDot discovered the coronavirus outbreak before the World Health Organization and the US Centers for Disease Control this past December. Here are some links:
https://www.wired.com/story/ai-epidemiologist-wuhan-public-health-warnings/
https://www.cnbc.com/2020/03/03/bluedot-used-artificial-intelligence-to-predict-coronavirus-spread.html
https://www.forbes.com/sites/simonchandler/2020/03/05/how-ai-will-prevent-the-next-coronavirus-pandemic/#2bbdf1054ac6